B.M. Jesdale1, S.A. Chrysanthopoulou1,2, C.E. Dubé1, Kate L. Lapane1
1. Department of Quantitative Health Sciences, University of Massachusetts Medical School, Worcester, MA; 2. Department of Statistics, Center for Statistical Sciences, Brown University, Schoolf of Public Health. Corresponding author: Kate L. Lapane, Albert Sherman Center 6th floor, Department of Quantitative Health Sciences, University of Massachusetts Medical School, 55 Lake Road North, Worcester MA 01655, USA, Email: Kate.Lapane@umassmed.edu, Phone: 508-856-8965, Fax: 508-856-8993
Jour Nursing Home Res 2018;4:36-41
Published online October 18, 2018, http://dx.doi.org/10.14283/jnhrs.2018.7
Abstract
Objectives: We estimated the impact of safe patient handling legislative efforts to reduce nursing home worker injuries, and examined potential impacts among specific nursing home types. Design: Difference-in-difference analysis. Setting: 2,034 nursing homes in 8 states enacting safe patient handling and movement legislation from 2004 to 2007 and 5,901 nursing homes in 36 comparator states. Measures: Reductions in reported work-related injuries and illnesses resulting in Days Away from work, Restricted job activities, or Transfer (DART) rates per 100 full time equivalents (FTE’s). Facility characteristics included size, profit orientation, chain membership, nursing staffing measures, and location (urbanicity). Results: Among nursing homes in 8 states that enacted legislation, there was a 23.5% decrease in mean DART rate from 7.53 per 100 FTE’s in the pre-enactment period (2002-2003) to 5.76 per 100 FTE’s in the post-enactment period (2008-2010) whereas in 36 comparator states, there was a 24.4% decrease in the mean DART rate, from 8.54 to 6.46 per 100 FTE’s. After adjustment for nursing home and aggregated resident characteristics, a difference-in-difference model showed that DART rates were similar in states with and without legislation (adjusted estimate: 1.03; 95% confidence interval: 0.96 to 1.11), with estimates similar across a range of nursing homes characteristics. Conclusions: The promise of enacting safe patient handling and movement legislation to reduce nursing home worker injuries has yet to be realized. In a context of rapidly declining injury rates, substantial financial incentives, other forms of assistance, and/or enforcement activities may be needed to improve the effectiveness of legislative initiatives.
Key words: Nursing homes, long-term care, staff injuries, occupational health, regulation.
Introduction
Worker injury rates in nursing homes are among the highest among occupational sectors in the United States (1). Although worker training alone appears to have little impact (2), comprehensive interventions at the nursing home level to reduce the burden of nurse injuries have reduced not only staff injuries, but also lost workdays, and workers’ compensation costs (3-8). Training and hiring replacement staff has high costs (9) as does losing seasoned staff to preventable injuries (10).
Numerous states have enacted legislation designed to reduce worker injuries in the health care sector, including nursing homes. The content and extent of these legislative efforts varies widely, from the funding of demonstration projects (Ohio, 2004) (11), to aspirational statements (Hawaii, 2005) (12). We have described these legislative efforts in detail elsewhere (13).
Declines in nursing home worker injury rates in selected nursing homes located in Ohio (14, 15) and New York (16) were seen after implementation of Safe Patient Handling and Movement (SPHM) legislation. Statewide, compensable injury rates among workers in hospitals in Washington state declined, but not more so than in Idaho, a neighboring state with no SPHM enactment (17). In a nationwide analysis, injury rates among health care and social assistance workers declined in most, but not all, states enacting SPHM legislation in the year following implementation (18). Aside from the Washington study (17), none of these analyses accounted for rapidly declining trends in injury rates in unaffected states (19).
We sought to estimate the effect of state-level SPHM legislation on nursing home worker injury rates, accounting for declining worker injury rates in the nursing home sector nationwide, and adjusting for potential shifts in resident characteristics over time. Further, we investigated whether the apparent effect of SPHM legislation would differentially affect nursing homes according to pre-specified nursing home characteristics, namely: bed size, profit orientation, chain membership, nurse:bed ratio, staff mix (ratio of registered nurses to other nursing staff), and urbanicity.
Methods
This study was approved by the Institutional Review Board at our institution.
Data sources
Appendix 1 reviews the data sources considered for conducting this study. We included private sector nursing homes not affiliated with a hospital and in operation throughout the study period (2002-2010) with identifiable data from three sources: the Centers for Medicare and Medicaid Services Provider of Service (POS) annual files (addresses, hospital affiliation, profit orientation, chain membership, bed size, the number of staff full time equivalents (FTEs), and workforce composition), the Occupational Health and Safety Administration’s OSHA Data Initiative (ODI) (annual nursing home-level injury rates, addresses), and the Minimum Data Set (MDS) (annual distribution of resident-level characteristics). Urbanicity was assigned to nursing homes using a six-level urbanicity code developed by the National Center for Health Statistics (20).
The ODI contains establishment-level, work-related injury and illness rates reported by work establishments, including nursing homes from 1996 to 2011. Government-owned nursing homes were usually exempt from reporting requirements, as were nursing homes with fewer than 10 employees. Seven states were waived from ODI reporting requirements for two or more consecutive years during our study period (Alaska, Arizona, Oregon, South Carolina, Virginia, Washington, Wyoming), and are not included in our analyses.
Collected by federal mandate in all Medicare/Medicaid certified homes, the MDS is a standardized resident assessment instrument completed at admission, quarterly, annually, readmission, and when there is a significant change in clinical status. The MDS collects a wide range of clinical and functional data, including height and weight of residents, mobility status, and functional capacity. We calculated quarterly aggregates of resident characteristics using MDS version 2.0 (1/1/2002 to 9/30/2010), then averaged these quarterly aggregates to obtain annual descriptors of resident characteristics.
Sample
We identified 11,491 nursing homes using the POS files. See Supplementary Table 2 and Appendix 2 for details regarding the matching procedures of the data sources. Eligible nursing homes were those that were open throughout 2002-2010, not government-owned, not hospital affiliated, and located in one of the 43 states or the District of Columbia (DC) that collected ODI data. The ODI does not contain a unique identifier for nursing homes, but includes self-reported name, address, and industrial sector codes which were used to match 10,584 (92.6%) of the nursing homes above to ODI data for at least one year. Of all eligible nursing homes collecting ODI data, 11,425 (99.4%) were successfully merged with their resident data in the MDS. To be eligible, information on more than 9 residents for a nursing home was required for each study year. To reduce missing data, we further restricted our sample to 7,935 nursing homes with all of the following: 1) ODI data for at least one year in the period from 2000 to 2004 (before any state enacted SPHM legislation); 2) ODI data for at least one year in the period 2008-2011 (after all states had enacted SPHM legislation); and 3) no more than 5 consecutive years of missing ODI data in 2002-2010. Of otherwise eligible nursing homes 69.5% were excluded (see Supplementary Tables 3 and 4 for a comparison of excluded nursing homes to the otherwise eligible sample).
Exposure periods
Eight states with ODI injury rate reporting enacted SPHM legislation between 2004 and 2007 (Hawaii, Maryland, Minnesota, New Jersey, New York, Ohio, Rhode Island, Texas). No states had enacted SPHM legislation before this period, and no others enacted SPHM legislation directed towards nursing homes before 2010. We defined three periods for legislation enactment in the SPHM legislation states: a pre-enactment period from 2002 to 2003, an enactment period from 2004 to 2007, and a post-enactment period from 2008 to 2010.
Outcome assessment
ODI data are reported as the DART rate (reported work-related injuries or illnesses resulting in Days Away from work, Restriction of job activities, or Transfer to another position, per 100 FTE’s). In general, nursing homes were required to report injury and illness rates every three years, with nursing homes reporting a high injury rate usually required to report again the following year. Following previous literature (21-23), we treated DART rates higher than 50 per 100 FTE’s (0.08% of reported values) as likely outliers, and re-set these to missing.
Imputation of missing values
Among the 7,935 nursing homes included in the 9 years of the analysis, 74.4% of nursing home-years were populated with reported ODI data. A relatively low injury and illness rate in the previous year strongly predicted missing data; of those with an observed DART rate under 5.00 per 100 FTE’s, 51.1% had missing data in the following year, compared to only 13.9% of those with an observed DART rate of 5.00 per 100 FTE’s or higher. Missing data increased over time, from 8.9% in 2002 to 50.5% in 2010. State-level missing data varied from 11.8% of nursing home-years in Maine to 47.2% in the District of Columbia. Missing data were modestly associated with some nursing home-level characteristics (see Supplementary Table 5).
We used multiple imputation to estimate the natural logarithm of DART rates from 1996 to 2011, in which injury rates of 0 were replaced with ln(0.5) (post-imputation exponentiated values of 0.5 or lower were set to 0). To restrict DART rate imputations to plausible values, an upper limit of ln(100) (twice the cut-point for re-assigning a reported value to missing) was applied. Details regarding the imputation method are provided in the Supplementary Imputation Appendix 3.
The analysis and imputation unit is the nursing home. For the imputation we used 16 measured variables potentially related to DART rates and missingness, including the region of the country (10 Medicare regions), the urbanicity of the nursing home’s county (6 categories), profit orientation in 2002 and 2010 (2 categories at each time point), and chain membership in 2002 and 2010 (2 categories at each time point). We also included the mean values across the 9 years for the number of beds, staffing levels, and nursing home-level aggregates of resident characteristics, including resident age, weight, and proportions of residents with clinical, demographic, and care-related factors. We also included the mean values across the 9 years for number of beds, staffing levels, and nursing home-level aggregates of resident characteristics including resident age, weight, and proportions of residents with clinical, demographic, and care-related factors. We produced 50 imputed datasets using PROC MI in SAS, version 9.4 (SAS Institute, Inc., Cary, NC), using the fully conditional specification (FCS) method with 100 burn-in imputations.
Analytic strategy
We estimated the impact of SPHM legislation on nursing home-level DART rates using the Generalized Estimating Equations (GEE) method to fit a Poisson model including status (SPHM legislation state or not), the three exposure periods, and an interaction between these terms. The exponentiated coefficient of the interaction term comparing the post-enactment period to the pre-enactment period is interpreted as the difference between the average injury rate (per 100 FTE’s) observed in enactment states relative to what would be expected if the same temporal trend had been held as in comparator states; a ratio greater than 1 indicates a higher injury rate than expected, and a ratio between 0 and 1 indicates a lower injury rate than expected. We weighted observations by the total number of FTE’s among full and part time employees in the nursing home in each year. We also adjusted this analytic model with a subset of the variables included in the imputation model (see footnote in Table 3).
To estimate the effect of SPHM legislation within subgroups of nursing homes, we repeated the analyses above after nesting the main effects within pre-specified nursing home characteristics, namely: bed size (under 100 beds, 100-299 beds, 300+ beds), profit orientation (yes, no), chain membership (yes, no), nurse:bed ratio (0.05 to 0.50, 0.50 to 0.70, 0.70 to 2.51), ratio of registered nurses to other nursing staff (0.01 to 0.15, 0.15 to 0.25, 0.25 to 2.59), and urbanicity (large metropolitan areas of 1 million or higher population, medium or small metropolitan areas of 100,000 to 999,999 population, or micropolitan and rural areas). We compared our findings with a complete-case analysis (no imputed values).
Sub-analyses
We conducted sub-analyses to further investigate the impact of legislation under alternate specifications: 1) a “high contrast” comparison restricting the SPHM states to the four enacting the most comprehensive forms of legislation (Maryland, Minnesota, New Jersey, Rhode Island), and excluding three states that enacted SPHM legislation after our study period; 2) a comparison of 7 SPHM legislation states (excluding Hawaii) to 18 states neighboring at least one SPHM legislation state; and 3) an analysis using a naïve single imputation method (linear interpolation from 2002-2010, or carry last observation forward after the last observed DART rate).
Results
Nursing homes in the 8 states with safe patient handling and movement (SPHM) legislation differed from those in the 36 comparator states in several respects (Table 1). Nursing homes in the states that enacted SPHM legislation were more frequently large homes (11% vs. 2% with 300 or more beds), for-profit in orientation (66% vs. 73%), part of a chain (43% vs. 58%), or located in a central county, large metropolitan area (33% vs. 20%).
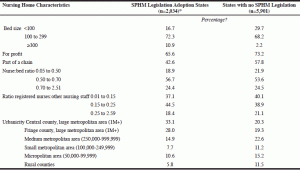
Table 1
Nursing Home Characteristics in 2002, by Safe Patient Handling and Movement (SPHM) Legislation Status
* Hawaii, Maryland, Minnesota, New Jersey, New York, Ohio, Rhode Island and Texas; † Percents are weighted by size of nursing home staff.
Table 2 shows that DART rates in SPHM legislation enacting-states declined from an average of 7.53 per 100 FTE’s in the pre-enactment period (2002-2003), to 6.50 per 100 FTE’s in the enactment period (2004-2007), and further to 5.76 per 100 FTE’s in the post-enactment period, an average drop of 1.77 work-related injuries and illnesses per 100 FTE’s. DART rates were, on average, higher in the comparator states, declining from 8.54 per 100 FTE’s in the pre-enactment period to 7.37 per 100 FTE’s in the enactment period to 6.46 per 100 FTE’s in the post-enactment period, an average drop of 2.08 per 100 FTE’s. Wide variation between homes is also apparent.

Table 2
Distribution of Injury and Illness Rates by Enactment Period and Safe Patient Handling and Movement (SPHM) Legislation Status
* DART rate: work-related injuries and illnesses resulting in Days Away from work, Restriction of job activities, or Transfer to another position, per 100 FTE’s per year. † Hawaii, Maryland, Minnesota, New Jersey, New York, Ohio, Rhode Island and Texas. ‡ Averages, medians, 25th and 75th percentiles are weighted by the staff size of the nursing homes. § During the pre-enactment period (2002-2003), no states had yet enacted SPHM legislation. All 8 SPHM states enacted legislation between 2004 and 2007. No states enacted SPHM legislation during the post-enactment period (2008-2010).
Table 3 shows crude and adjusted estimates for the effect of enacting SPHM legislation on DART rates. In the unadjusted analysis, we estimate that DART rates were 6% higher in SPHM legislation-enacting states in the post-enactment period than they would have been had they followed the same secular trend as seen in comparator states [Rate Ratio (RR)=1.06, 95% Confidence Interval (CI): 0.93 to 1.20]. After adjustment for a wide range of static and time-varying nursing home and aggregated resident characteristics, our estimates were similar: DART rates were 3% higher than expected [RR=1.03, 95% CI: 0.96 to 1.11] . Table 3 also shows difference-in-difference estimates for various subsets of nursing homes. These subsets show similar patterns to those in the whole population of nursing homes studied, especially after adjustment.
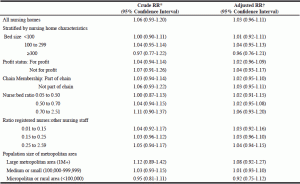
Table 3
Difference in difference estimates for the impact of safe patient handling and movement (SPHM) legislation
* Ratio of work-related injury and illness rates during the post-enactment period (2008-2010) to the pre-enactment period (2002-2003) among SPHM states relative to the ratio during the post-enactment period to the pre-enactment period in states with no SPHM legislation. Pooled across 50 multiply imputed datasets, weighted by the staff size of the nursing homes, and with a generalized estimating equation specification (m-dependent correlation matrix) to account for repeated measures for each nursing home. 95% confidence intervals generated from model-based standard error estimates; † As above, and adjusted for state, year, urbanicity, calendar year, and the following time-varying covariates: profit orientation, chain membership, nursing home bed size, nurse:bed ratio, ratio of registered nurses to other nursing staff, mean resident weight, mean resident age, percent of residents with: severe activities of daily living limitations, require mechanical lifting, resist care and are not easily modified, have conflicted relationships with staff, restrained, loss of movement in one or both legs, had fallen in the previous 30 days and/or had a hip fracture in the previous 180 days, had dementia and/or Alzheimer’s, had bipolar depression, had a high grade (2-4) pressure ulcer, used antipsychotics in the previous week, and resident proportion Asian, proportion Black, and proportion Hispanic residents.
Complete-case (Supplementary Table 9) and sub-analyses resulted in similar results (see Supplementary Tables 7, 8, and 10); restricting the analysis to a “high contrast” comparison between 4 states that enacted comprehensive SPHM legislation and after excluding three states from the comparator group that enacted SPHM after our observation period (Supplementary Table 7); restricting the comparator group of states to those with at least one SPHM legislation-enacting neighbor (Supplementary Table 8); or using a naïve single imputation model (Supplementary Table 10).
Discussion
These findings were contrary to our expectation. We hypothesized that injury rates in SPHM legislation-enacting states would have declined more so than comparator states. Several alternate explanations for these findings should be considered. Publicity around the enactment of SPHM legislation may have increased the proportion of worker injuries and illnesses reported to employers, and/or have encouraged employers to more faithfully record reported worker injuries and illnesses. If this were the case, then a lower rate or proportion of serious injuries might be expected in the SPHM legislation-enacting states. Unfortunately, we did not have the data to evaluate this. Likewise, several states required nursing homes to enact written SPHM policies, which may have increased worker knowledge and use of reporting mechanisms increasing reported injuries which may have been previously unreported. We do not have specific evidence to support or refute these possibilities.
Another possibility arises from the nature of the SPHM legislation itself. In most cases, these state-wide enactments included no appropriations to assist nursing homes with enacting SPHM policies, purchasing lift equipment, or even to offset the paperwork burden of compliance with the new regulations. Furthermore, most included no compliance enforcement provisions (13). Although our analysis of states enacting more comprehensive legislation produced similar results to our overall findings, these legislative efforts may have fallen short of providing sufficient incentives or disincentives to motivate lasting, integrated, efforts to reduce worker injuries. Several studies have found that provision of lift equipment alone or provision of worker training alone fail to produce a lasting impact on worker injury rates. A multicomponent approach to reducing worker injuries appears to be required within individual nursing homes or hospitals (3, 6, 8, 24, 25) and within an entire healthcare delivery system (26).
While convincing evidence of efficacy exists for the impact of legislative actions to generate change in nursing homes in some settings (27, 28) it is not unusual for efforts to assess legislative efficacy to fail to document substantive change (29). For example, state-level restrictions on feeding tube use in severely cognitively impaired residents appear to have a small impact on preventing their use (30, 31) while legislation designed to increase staffing by qualified social workers has largely been circumvented, failing to produce improvements in quality of care (32), and legislation to improve overtime payments for nurses appears to paradoxically have reduced care quality by encouraging nursing homes to substitute contract workers for experienced staff (33). The extent of state efforts to enforce legislation appears to have a sizeable impact on the efficacy of the legislation as well (34), as does the infrastructure capacity of legislative bodies themselves (35). It is also possible that the legislative enactments intended to reduce worker injuries that were included in our analysis were not sufficiently enforced to produce marked reductions.
Finally, these legislative efforts occurred during a period of rapidly declining worker injury rates, both in nursing homes (19), and in the nation’s workplaces as a whole (36). Overlapping legislative efforts, shifts in worker compensation systems, and a growing focus on occupational injuries in general (37, 38) may have affected nursing homes in states that did not enact SPHM legislation during this time period.
Conclusion
The promise of enacting safe patient handling and movement legislation to reduce nursing home worker injuries has yet to be realized. In a context of rapidly declining worker injuries nationwide, 36 states enacting SPHM legislation had somewhat slower declines in reported worker injury rates than comparator states. Furthermore, estimates for a range of nursing home subtypes failed to demonstrate markedly different responses. National or state-level legislation including substantial financial incentives, other forms of assistance, and/or enforcement activities may improve the efficacy of legislative initiatives. Wider enactment of these more comprehensive laws would be required to evaluate the potential for greater efficacy.
Acknowledgements: This study was funded by a grant from the National Institute For Occupational Safety And Health.
Conflict of Interest: None
Ethical Standard: Approval by the University Massachusetts Medicals School Institute at Review Board.
References
1. Bureau of Labor Statistics, United States Department of Labor. Employer-Reported Workplace Injuries and Illnesses, 2016. Summary Table 1. Available from: https://www.bls.gov/web/osh/summ1_00.xlsx. Accessed 2017;19 Jan 2018.
2. Fragala G, Ponatani Bailey L. Addressing occupational strains and sprains: musculoskeletal injuries in hospitals. AAOHN J 2003;51:252-259. PMID: 12846458
3. Stenger K, Montgomery LA, Briesemeister E. Creating a culture of change through implementation of a safe patient handling program. Crit Care Nurs Clin N Am 2007;19:213-222. DOI: 10.1016/j.ccell.2007.02.007
4. Lipscomb HJ, Schoenfisch AL, Myers DJ, et al. Evaluation of direct workers’ compensation costs for musculoskeletal injuries surrounding interventions to reduce patient lifting. Occup Environ Med 2012;69:367-372. DOI: 10.1136/oemed-2011-100107
5. Nelson A, Matz M, Chen F, et al. Development and evaluation of a multifaceted ergonomics program to prevent injuries associated with patient handling tasks. Int J Nurs Stud 2006;43:717-733. DOI: 10.1016/j.ijnurstu.2005.09.004
6. Lim HJ, Black TR, Shah SM, et al. Evaluating repeated patient handling injuries following the implementation of a multi-factor ergonomic intervention program among health care workers. J Safety Res 2011;42:185-191. DOI: 10.1016/j.jsr.2011.05.002
7. Li J, Wolf L, Evanoff B. Use of mechanical patient lifts decreased musculoskeletal symptoms and injuries among health care workers. Inj Prev 2004;10:212-216. DOI: 10.1136/ip.2003.004978
8. Schoenfisch AL, Lipscomb HJ, Pompeii LA, et al. Musculoskeletal injuries among hospital patient care staff before and after implementation of patient lift and transfer equipment. Scand J Work Environ Health 2013;39:27-36. DOI: 10.5271/sjweh.3288
9. The HSM Group, Ltd. Acute care hospital survey of RN vacancy and turnover rates in 2000. J Nurs Admin 2002;9:437-439. PMID: 12360112
10. Hatcher B, Bleich MR, Connolly C, et al. Wisdom at Work: The Importance of the Older and Experienced Nurse in the Workplace. Robert Woods Johnson Foundation, 2006. Accessed 19 Jan 2018. Available at: https://www.rwjf.org/content/dam/farm/reports/reports/2006/rwjf13773
11. Ohio General Assembly. (2004). House Bill 67, 126th General Assembly. An Act to amend sections 121.08, 4121.12, 4121.121, 4121.37, 4123.511, 4167.02, 4167.06, 4167.07, 4167.08, 4167.09, 4167.10, 4167.11, 4167.12, 4167.14, 4167.15, 4167.16, 4167.17, 4167.19, and 4167.27, to enact section 4121.48, and to repeal section 4167.18 of the Revised Code to transfer the Public Employees Risk Reduction Program and the Occupational Safety and Health Act On-site Consultation Program from the Department of Commerce to the Bureau of Workers’ Compensation, to make appropriations for the Bureau of Workers’ Compensation for the biennium beginning July 1, 2005, and ending June 30, 2007, and to provide authorization and conditions for the operation of the Bureau’s programs. Accessed 25 Oct 2017. Available at: http://archives.legislature.state.oh.us/BillText126/126_HB_67_EN_N.pdf
12. Hawaii House of Representatives. (2006). House Concurrent Resolution 16. Requesting Appropriate Safeguards be Instituted in Health Care Facilities to Minimize the Occurrence of Musculoskeletal Injuries Suffered by Nurses. Accessed 11 May 2016. Available at: http://www.capitol.hawaii.gov/session2006/Bills/HCR16_.pdf
13. Lapane KL, Dubé CE, Jesdale BM. Worker injuries in nursing homes: is safe patient handling legislation the solution? J Nurs Home Res 2016 2:110-117.
14. Park RM, Bushnell PT, Bailer AJ et al. Impact of publicly sponsored interventions on musculoskeletal injury claims in nursing homes. Am J Ind Med 2009 52:683-697.
15. Ohio Bureau of Workers’ Compensation. Ergonomics Best Practices for Extended-Care Facilities. (undated). Accessed 13 Jan 2014. Available at: https://www.bwc.ohio.gov/downloads/brochureware/publications/ExtCareSafeGrant.pdf
16. Safe Patient Handling Workgroup, New York State Department of Health. Report to the Commissioner of Health. (undated). Accessed 31 May 2016. Available at: https://www.health.ny.gov/statistics/safe_patient_handling/docs/sph_report.pdf
17. Silverstein B, Howard N, Adams D, Schurke J. Implementation of Safe Patient Handling in Washington State Hospitals. Olympia, WA: Washington State Department of Labor and Industries, 2011. Accessed 13 Jan 2014. Available at: http://www.lni.wa.gov/Safety/Research/Files/SafePatientHandlingRpt2010.pdf
18. Wrightson K, Lincoln T, Harley S, Sanoian B. Uplifting an Industry? State-Based Safe Patient Handling Laws Have Yielded Improvements But Are Not Adequately Protecting Health Care Workers. Washington, DC: Public Citizen’s Congress Watch, 2105. Accessed 31 May 2016. Available at: https://www.citizen.org/sites/default/files/part-three-state-health-care-worker-safety-laws-uplifting-industry.pdf
19. Bureau of Labor Statistics, United States Department of Labor. Employer-Reported Workplace Injuries and Illnesses, 2016. Washington, DC: United States Department of Labor, 2017. Accessed 19 Jan 2018. Available at: https://www.bls.gov/news.release/pdf/osh.pdf
20. Ingram DD, Franco SJ. 2013 NCHS urban-rural classification scheme for counties. National Center for Health Statistics. Vital Health Stat 2014;2(166).
21. Groenewold M. County-level demographic and socioeconomic correlates of high establishment-level occupational injury and illness rates. 2013 Annual Council of State and Territorial Epidemiologists Conference, Pasadena. Accessed 30 Jan 2014. Available at: https://cste.confex.com/cste/2013/webprogram/Paper1511.html
22. Mendeloff J, Gray WB, Haviland AM, Main R, Xia J. An evaluation of the California Injury and Illness Prevention Program. RAND Health Q 2012;2:16
23. Neff RA, Curriero FC, Burke TA. Just in the wrong place? Geographic tools for occupational injury/illness surveillance. Am J Ind Med 2008;51:680-690.
24. Dawson AP, McLennan SN, Schiller SD et al. Interventions to prevent back pain and back injury in nurses: a systematic review. Occup Environ Med 2007;64:642-650.
25. Yassi A, Cooper JE, Tate RB et al. A randomized controlled trial to prevent patient lift and transfer injuries of health care workers. Spine 2001;26:1739-1746.
26. Powell-Cope G, Toyinbo P, Patel N et al. Effects of a national safe patient handling program on nursing injury incidence rates. J Nurs Adm 2014;44:525-534.
27. Shorr RI, Fought RL, Ray WA. Changes in antipsychotic drug use in nursing homes during implementation of the OBRA-87 regulations. JAMA 1994;271:358-362.
28. Teno JM, Branco KJ, Mor V et al. Changes in advance care planning in nursing homes before and after the Patient Self-Determination Act: report of a 10-state survey. J Am Geriatr Soc 1997;45:939-944.
29. Wiener JM. An assessment of strategies for improving quality of care in nursing homes. Gerontologist 2003;43:19-27.
30. Teno JM, Mor V, DeSilva D et al. Use of feeding tubes in nursing home residents with severe cognitive impairment. JAMA 2002;287:3211-3212.
31. Ahronheim JC, Mulvihill M, Sieger C et al. State practice variations in the use of tube feeding for nursing home residents with severe cognitive impairment. J Am Geriatr Soc 2001 49:148-152.
32. Bowblis JR, Smith AC. Occupational licensing of social services and nursing home quality: a regression discontinuity approach. Stanford, CA: Social Science Research Network, 2018. Accessed 1 Feb 2018. Available at: https://ssrn.com/abstract=3096268
33. Lu SF, Lu LX. Do mandatory overtime laws improve quality? Staffing decisions and operational flexibility of nursing homes. Management Sci 2016;63:3566-3585.
34. Bowblis JR, Crystal S, Intrator O, Lucas JA. Response to regulatory stringency: the case of antipsychotic medication use in nursing homes. Health Econ 2012;21:977-993.
35. Boehmke FJ, Shipan CR. Oversight capabilities in the states: are professionalized legislatures better at getting what they want? State Politics Policy Q 2015;15:366-386.
36. Bureau of Labor Statistics, United States Department of Labor. (2017). Employer-Reported Workplace Injuries and Illnesses, 2016. Accessed 19 Jan 2018. Available at: https://www.bls.gov/news.release/pdf/osh.pdf
37. Bhushan A, Leigh JP. National trends in occupational injuries before and after 1992 and predictors of workers’ compensation costs. Pub Health Rep 2011;126:625-634.
38. Conway H, Svenson J. Occupational injury and illness rates, 1992-96: why they fell. Monthly Lab Rev 1998;121:36-58.