L.D. Kimmey1, S.C. Stearns2
1. Researcher, Mathematica Policy Research, Washington, DC, USA; 2. Department of Health Policy and Management, The University of North Carolina at Chapel Hill, Chapel Hill, NC. Corresponding author: Laura D. Kimmey, 1108 Chancellors Ridge Drive, Durham, NC, 27713, Phone: 202-264-3470, Fax: 202-863-1763, lkimmey@mathematica-mpr.com
Abstract
Background: Nursing home staffing characteristics have long been hypothesized to affect quality of care and resident outcomes. The current literature lacks a solid understanding of the magnitude of the effects that sufficient staff, low turnover, and good nursing oversight can have on outcomes that are important to residents and payers. Knowledge of the magnitudes of these effects is essential to evaluating future directions for long-term care research and policy. Objectives: This study estimates the effects of staffing components on the following resident outcomes: pain, pressure ulcers, and restraint use; falls; and emergency department use and hospitalizations. Design: Prior estimates of the relationship between staffing components and resident outcomes suffer from limitations including small samples and use of facility rather than resident-level data. This study allows for joint and non-linear effects of staffing characteristics on undesirable outcomes. We allowed for non-linear relationships by including squared measures of staffing hours per resident day and interactions between staffing levels and retention by type of staff. Our final models used logistic regression with sample weights calculated by the National Center for Health Statistics. Setting: Residents and facilities participating in the 2004 National Nursing Home Survey. Participants: The 2004 National Nursing Home Survey sampled 14,017 residents at 1,500 of the 16,628 nursing homes in the United States; facility and resident response rates were 81% and 96%, respectively. Exclusions for various criteria and missing data result in an analysis sample of 10,043 residents at 954 facilities. Measurements: We assessed three dichotomous outcome measures. The first measure combined reports of pain in the week prior to the survey, pressure sores at the time of the survey, or whether restraints were ever used for the resident. The second measure was a report of any fall in the 30 days prior to the survey, and third was any emergency department visits or hospital admissions in the previous 90 days. Key explanatory variables reflected staffing levels (ratios of staff hours per patient day and registered nurse bedside hours), retention (proportion of staff employed at least one year) and mix (ratio of registered nurses to licensed practical nurses and certified nursing assistants). Results: Moving from very poor to very good staffing improves predicted outcomes in plausible ways: pain, pressure sores or restraint use decline with higher certified nursing assistant hours and retention, 30-day fall rates decline with greater registered nurse oversight, and emergency department /hospital use decline with more registered nurse bedside hours. Conclusions: Both the absolute and relative effects of moving from very poor to very good staffing are limited in magnitude, demonstrating that broader approaches to improving nursing home quality are needed to ensure satisfactory outcomes for nursing home residents. Additional research is needed to better understand what components of facility administration and operation are most strongly associated with resident outcomes.
Key words: Quality, outcomes, turnover, staffing.
Introduction
Nursing home staffing characteristics have long been hypothesized to affect quality of care and resident outcomes. Nursing home staff with direct resident contact include certified nursing assistants (CNAs), licensed practical nurses (LPNs), and registered nurses (RNs). In the United States, CNAs assist in providing nursing care, including taking vital signs and helping residents with activities of daily living such as bathing (1). LPNs can perform the same duties as CNAs, and additional duties including giving medicine and documenting patient condition (1). RNs can perform the same duties as LPNs, and additional duties include giving intravenous medications and performing physical assessments (1). Staffing characteristics encompass a range of measures including the type of staff used, staff to resident ratios, staffing stability (i.e., turnover), and ratios among different types of staff (e.g., RN oversight). Detailed literature reviews summarize prior research on the associations of quality of care with staffing levels and stability (2-4). These literature reviews find moderate support for a positive association between staffing levels and quality of care and a negative association between turnover and quality of care. The literature suggests that both extremely high and extremely low turnover have deleterious effects (5, 6). One study found statistically significant non-linear and interactive effects of staffing levels and turnover, but the authors did not estimate the size of the improvements in outcomes associated with better staffing characteristics (7, 6).
The current literature lacks a solid understanding of the magnitude of the effects that sufficient staff, low turnover, and good nursing oversight can have on outcomes that are important to residents and payers. Knowledge of the magnitudes of these effects is essential to evaluating future directions for long-term care research and policy. Use of LPNs and CNAs has increased in recent years, while RN use and oversight have decreased (8). State staffing regulations in the US may ensure staffing at certain levels that may help ensure adequate care, but meeting those regulations may not be sufficient to maximize outcomes. Recent calls for efforts to improve quality of care at nursing homes have focused on broader approaches such as improving the quality of the long-term care workforce (9), encouraging broader quality improvement and culture-change initiatives in nursing homes (10), or a series of innovative interventions within nursing homes themselves (11).
Our study extends the existing literature and contributes to this debate by: (1) examining independent and joint effects of staffing characteristics by type of staff on undesirable outcomes among nursing home residents; and (2) using predictions to estimate the potential for improvements in outcomes by increasing staffing levels, stability or professional mix.
Methods
Conceptual and Empirical Model
The conceptual model is based on a framework in which staffing levels, staff stability, and professional staff mix are hypothesized to be related to resident outcomes. Staffing levels may be related to outcomes through impacts on the quantity of care provided, as high staff to resident ratios may be necessary to provide sufficient time for caregivers to perform tasks. High turnover or low retention may reduce quality of care by increasing employees’ workload, increasing the number of inexperienced workers, diverting money from resident care to staff replacement and retraining, and reducing employees’ familiarity with individual residents’ needs. Greater levels of RN oversight of other direct care staff may enhance early targeting of potential problems or facilitate high quality care by other direct care staff.
We use resident observations to estimate outcomes as a function of staffing variables, other facility characteristics, and resident characteristics. Our approach avoids some of the limitations of prior studies such as the use of facility rather than resident-level outcome measures, small or geographically unrepresentative samples, or failure to analyze staffing levels and turnover jointly for multiple types of staff (4, 7, 12-16).
Data
Data are from the 2004 National Nursing Home Survey (NNHS) conducted by the US National Center for Health Statistics (NCHS). Although the survey is a decade old, it is the most recent US national survey that links facility-level staffing measures with resident outcomes. Because the analyses required restricted data to merge the resident and facility files, the data were constructed by NCHS and accessed through the Triangle Census Research Data Center. The 2004 NNHS surveyed 13,507 residents from 1,174 facilities in the US. Since our focus was on nursing home care for older adults, we excluded residents less than age 55.
Resident Outcomes Measures
We assessed three dichotomous outcome measures. The firWe assessed six dichotomous outcome measures. The first measure combined reports of pain in the week prior to the survey, pressure sores at the time of the survey, or whether restraints were ever used for the resident. We chose a composite measure because combining these important, commonly used variables increased the power of the analysis. The second through fourth measures consisted of separate measures of pain, pressure sores or restraints. The fifth measure was a report of any fall in the 30 days prior to the survey; we analyzed falls for residents who were in the facility for at least 30 days (90% of the full sample). Finally, potentially undesirable heath service use was measured as any emergency department (ED) visits or hospital admissions in the 90 days prior to the survey. Given the current interest in reducing hospital readmissions for nursing home residents, especially potentially avoidable ED visits and hospitalizations by nursing home residents (17-19), we analyzed the combined ED/hospitalization measure for the full sample even though some residents may not have been in the nursing home for a full 90 days.
Facility Staffing Characteristics
Key explanatory variables reflected staffing levels, retention and mix. Ratios of staff hours per resident day (HPRD) were constructed separately for RNs, LPNs, and CNAs; these measures included permanent facility staff and agency staff. We also used a measure of RN bedside hours since RNs may spend substantial time on administrative duties. We used retention (proportion of staff employed at least one year) rather than turnover because estimates of turnover vary widely due to differences in measurement (2, 20). We measured professional staff mix as the ratio of RNs to LPNs and CNAs multiplied by 100, since greater use of RNs may signal greater oversight of staff and has been associated with lower hospitalization rates among assisted living residents (21). We allowed for non-linear relationships by including squared measures of RN, LPN, and CNA HPRD and interactions between staffing levels and retention by type of staff..
Resident and Facility Control Variables
Resident controls included age, race, gender, marital status at admission, payment sources in the month prior to the survey, and the following functional and cognitive status indicators: health status measures (feeding tube, whether the resident left the bed in the prior week, impairment in daily decision-making, presences of indicators of depression and how easily they are altered, and behavioral symptoms), number of medications, residence in Alzheimer’s/dementia unit, length of stay, and comorbidity indicators (congestive heart failure, valvular disease, pulmonary circulation, peripheral vascular, hypertension, paralysis, neurological condition, chronic obstructive pulmonary disease, diabetes, hypothyroidism, renal failure/acquired immune deficiency syndrome, liver disease, ulcer, cancer, arthritis, blood disease, obesity, weight loss, electrolytes, deficiency anemia, alcohol/drug, psychoses and depression). Facility-level controls included indicators of bed size, occupancy, percent of residents that were self-pay, and whether the facility was for-profit, part of a chain, had a geriatric specialist, assigned CNAs to the same residents, or used any agency staff.
Statistical Methods
Our final models used logistic regression with adjustments to the coefficient estimates for the complex survey structure of the data and sample weights calculated by NCHS. We used complete case analysis, so we further adjusted the sample weights to account for observations dropped due to missing data. The inclusion of squared and interacted variables precludes direct interpretation of the regression coefficients in non-linear models, so we used regression coefficients to predict the effect of changes in staffing. Given concerns about bias from omitted variables such as management quality as well as the cross-sectional nature of the data, all estimated effects should be interpreted as associations rather than causal estimates.
As noted earlier, prior studies have often found relatively modest marginal effects of small improvements in staffing. Our goal was to estimate the degree of reduction in poor outcomes that could be achieved by going from very low staffing to very high staffing in a model that allows for non-linear relationships and interactive effects between staffing measures. More specifically, our models allowed for the effects of increases in staffing levels on outcomes to be greater at low levels of staffing than at high staffing levels and to be different in facilities with high versus low retention. We simulated the effect of going from very low staffing (10th percentile) to very high staffing (90th percentile) staffing based on distributions of the staffing measures in the data. That is, using estimated coefficients from the regression, we simulated the expected level of outcome for a given staffing measure as if all facilities were in the 10th percentile for staffing, and we then simulated the expected level of outcome as if all facilities were in the 90th percentile. This wide range of values for the simulation allowed us to estimate the greatest feasible impact of changes in staffing measures on outcomes, although facility decisions or policy interventions (e.g., minimum staffing levels) would likely involve more modest changes in the measures.
Results
Table 1 provides weighted descriptive statistics for survey residents and the final sample of 10,043 residents based on complete case analysis. Approximately 21% of residents were missing data on one of the model variables; the last column of Table 1 contains the survey-weighted means, with weights adjusted for missing data. Thirty-five percent of the sample had pain, a pressure ulcer or restraint use. Any restraint use (6.8%) was less common than pressure ulcer at the time of the survey (10.6%) or pain in the last week (22.3%). Slightly less than 15% of residents who had been in the facility for 30 days reported a fall in the last 30 days, and 10.6% of the sample had an ED visit or hospitalization in the prior 90 days.
* The weights for the last column were adjusted for factors associated with missing data; † Additional resident control variables included health status measures (feeding tube, whether the resident left the bed in the prior week, impairment in daily decision-making, presences of indicators of depression and how easily they are altered, and behavioral symptoms), number of medications, residence in Alzheimer’s/dementia unit, length of stay, and Elixhauser comorbidity indicators (CHF, valvular disease, pulmonary circulation, peripheral vascular, hypertension, paralysis, neurological condition, COPD, diabetes, hypothyroidism, renal failure/AIDS, liver disease, ulcer, cancer, arthritis, blood disease, obesity, weight loss, electrolytes, deficiency anemia, alcohol/drug, psychoses and depression); ‡ Additional facility control variables included: beds squared, metropolitan status (metro, micro, small or rural), whether the facility had a geriatric specialist, whether the facility assigned CNAs to the same residents, whether the facility used any agency staff, and number of residents that were self-pay.
Most residents who were dropped due to missing data did not have facility staffing data. The values in the last column are very similar to the statistics for the original sample, which implies that residents who were dropped were similar to the final sample in many respects. For nearly all of the outcomes, resident control variables, and facility control variables, the absolute difference between the original sample and the final reweighted sample was less than 1 percentage point and/or the relative difference was less than 5%. There were three exceptions: (1) residents in the final reweighted sample were 3.1 percentage points (12.2%) less likely to have pain than the full sample; (2) residents in the final sample were 2.1 percentage points (21.6%) more likely to be black than the full sample; and (3) mean bed size at facilities included in the final sample was 31 beds (28.1%) higher than in the full sample.
Table 2 shows original and final reweighted analysis file statistics for the facility staffing measures, with the mean and 10th and 90th percentile values provided. For example, based on data from the 954 facilities used in the analysis, facilities reported a mean CNA retention rate (% of staff working at the same facility for at least a year) of 62.7%, with values of 33.0% at the 10th percentile and 87.0% at the 90th percentile. Mean retention rates were only slightly higher for LPNs and RNs, though the range of values for the 10th and 90th percentiles was greater.
Table 3 shows the predicted results for each outcome measure at very low (10th percentile) and very high (90th percentile) values of the staffing measures with 95% CIs for each estimate. (Full regression results are available upon request.) The interactions between HPRD and retention required that predictions for those measures were done jointly. While some of the estimated differences in predicted outcomes were large relative to the scale of the measure, many CIs were wide and overlapped substantially. The last column in Table 3 provides the estimated difference between the estimates if the CIs for the 10th and 90th percentile staffing measure estimates did not overlap (i.e., if the estimates were statistically different) or the direction of the effects that had overlapping CIs. Predictions from increasing staffing measures from the 10th percentile to the 90th percentile (holding everything else constant) showed that:
• Increased CNA HPRD and retention was associated with a 0.114 reduction in the likelihood of pain, pressure sore, or restraint use (27.0% reduction from the prediction based on the 10th percentile of the staffing measures);
• Increased CNA HPRD and retention was associated a 0.092 reduction in the likelihood of pain in the week before the survey (32.7% reduction from the prediction based on the 10th percentile of the staffing measures);
• Increased LPN HPRD and retention was associated with a 0.049 increase in the likelihood of pressure sore at the time of the survey (59.0% increase from the prediction based on the 10th percentile of the staffing measures);
• Increased professional mix was associated with a 0.042 reduction in the likelihood of a fall in the prior 30 days (27.6% reduction from the prediction based on the 10th percentile of the staffing measure); and
• Increased RN bedside HPRD was associated with a 0.022 reduction in the likelihood of ED visits or hospitalizations in the prior 90 days (19.3% reduction from the prediction based on the 10th percentile of the staffing measure).
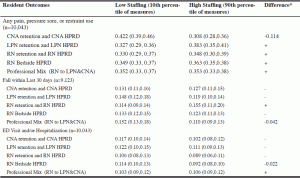
Table 3 Predicted Resident Outcomes and Association of Changes in Staff Measures with Outcomes (Logistic Regression Model)
* Numerical estimates are provided in cases where the predicted probability of the outcome is statistically significantly different at p<0.05 for the 90th percentile compared to the 10th percentile. Figure 1 shows the estimated values and the non-overlapping 95% confidence intervals for these estimates. The direction of the effect is indicated for all other cases that were not significant at p<0.05.
Other associations between staffing and outcomes in Table 3 were not statistically significant. Figure 1 provides a graphical depiction of the statistically significant results. The figure shows that the association between a simulated increase from very low to very high CNA staffing and pain, pressure sore, or restraint use as a composite as well as pain as an outcome measure by itself were larger in magnitude than the associations between (1) professional mix and falls and (2) RN bedside care and ED use or a hospitalization. In addition, the CIs for the statistically significant associations between CNA staffing and either the composite outcome or pain alone were far from overlapping, suggesting that those estimated associations may be quite statistically robust. The only change we identified that was not in support of improved outcomes from greater staffing was the increase in pressure sores associated with higher LPN HPRD and retention. This finding could be a statistical artifact (i.e., by assessing a number of different outcomes in relation to a number of explanatory variables, we would expect to find by chance associations that are not in the hypothesized direction five percent of the time). Alternatively, our controls for patient case mix may not be sufficient to control for the fact that when a greater proportion of residents have conditions that make them prone to pressure sores, facilities may hire more staff to improve care.
Discussion
Improving the quality of care for nursing home residents isWe demonstrate that the effects of very large changes in staffing are modest and still leave substantial room for improvement in outcomes. Specifically, the results suggest that efforts to improve nursing home quality of care and resident outcomes may require an approach much broader than simply increases in nursing home staffing levels or improvement in staff retention alone.
Improving the quality of care for nursing home residents is a critical objective given the increases in demand for nursing home care that are likely to accompany the upcoming demographic changes as baby boomers age. Important goals include not only improving the residents’ quality of life but also ensuring more rational use of limited health care resources by reducing unnecessary hospitalizations among nursing home residents. This analysis found statistically significant effects for one dimension of staffing for each outcome studied except restraint use, and the pathways for the estimated effects that were significant are largely plausible from a conceptual standpoint. For example, greater time spent by CNAs with residents may be the most critical factor for ensuring that pain is treated, and the magnitude of the association between CNA staffing and the outcome was even larger (in absolute value) for the composite measure which also included pressure sore and restraint use. Similarly, greater oversight by RNs (i.e., higher rates of RN to LPNs and CNAs) may be crucial for preventing falls. Finally, higher levels of time spent by RNs in providing bedside care may help reduce ED or hospital use by nursing home residents. The lack of significant relationships between higher LPN staffing and improved resident outcomes—and the one statistically significant effect, which was a positive association between a simulated increase in LPN staffing measures and the likelihood of having pressure sore—is similar to other work which has found fewer (and weaker) relationships between LPN staffing and outcomes than for CNA and RN staffing (22).
More importantly, however, the results demonstrate that higher staffing levels, retention or professional mix alone are insufficient to wholly transform nursing home care quality, and that beneficial effects on different outcomes may be largely due to certain aspects of staffing. While elimination of all poor outcomes is likely an infeasible and prohibitively expensive goal, the results indicate that regulation of staffing levels will not, by itself, achieve quality outcomes. As shown in Figure 1, an estimated 30% of residents may still have uncontrolled pain, pressure sore or restraint use even at very high levels of CNA staffing and low CNA turnover. In an analysis of the 2004 NNHS, Caffrey (17) showed that among the 8% of U.S. nursing home residents who had an ED visit in the past 90 days, 40% had a potentially preventable ED visit. Some nursing home residents will undoubtedly have appropriate and non-avoidable reasons for hospitalization, but Table 3 and Figure 1 in our analysis show that a simulated improvement from the 10th to 90th percentile of RN bedside HPRD only resulted in a 19.3 percentage point reduction to a predicted rate of combined ED visits and hospitalizations of 9.2%. It is possible that other potentially avoidable ED/hospital visits must be targeted through other approaches, such as expanded capabilities within nursing homes to assess, monitor and manage a wider range of patient conditions or more involvement with primary care providers (23).
The empirical approach is subject to several limitations. Because the NNHS is a cross-sectional sectional survey rather than a longitudinal data set with within-facility variation in staffing levels, the analysis was not able to control for unobserved facility characteristics that could affect both staffing characteristics and resident outcomes. Therefore, the effects estimated in this study are associations rather than causal effects. The estimation would be improved if longitudinal resident data were available that allowed within-facility variation in staffing over time. Such data would allow for facility fixed effects that could help eliminate bias from unobserved time-invariant facility characteristics in estimating the relationship between staffing characteristics and resident outcomes. For example, the finding that higher levels of LPN HPRD and retention were associated with greater levels of pressure sores may be due to facility-specific differences in case mix that were not sufficiently controlled with the patient level case mix measures used in the regressions. Another concern pertains to the fact that missing data on model variables caused about 21% of the original sample to be excluded from the complete case analysis. However, our adjustments to the sample weights should reduce or avoid bias from observed factors associated with missing data.
The important question for policy, however, is whether improvements can come through traditionally targeted approaches such as regulations regarding staffing levels or whether broader system-wide changes are needed (18). The analysis is not able to identify the important changes that are needed in the nursing home industry to elicit better outcomes. Current efforts to improve nursing home outcomes and quality advocate addressing other types of staffing-related changes, such as better education and training of the long-term care labor force [9] or a range of quality improvement approaches such implementing consistent assignment, using team approaches to care, or system-level processes like total quality management (10). Focusing long-term care research and policy on broader approaches may result in larger improvements in nursing home quality of care and resident outcomes than the traditional but relatively narrow focus on measures such as staffing levels and retention.
Funding: This work was supported by: the National Institute on Aging and the Demography and Economics of Aging Research Program at the Carolina Population Center (Grant 5-P30-AG024376); the National Institute on Aging through the Carolina Program for Health and Aging Research at the University of North Carolina Institute on Aging (2T32AG000272-06A2 to LDK); and the Agency for Healthcare Research and Quality through the Cecil G. Sheps Center for Health Services Research (5T32HS0000322 to LDK).
Acknowledgements: This research was conducted while the authors were Special Sworn Status researchers of the U.S. Census Bureau at the Triangle Census Research Data Center. The National Center for Health Statistics provided the merged data set. This paper has been screened to ensure that no confidential data are revealed. The findings and conclusions in this paper are those of the authors and do not necessarily represent the views of the Triangle Census Research Data Center, the National Center for Health Statistics, or the Centers for Disease Control and Prevention.
Disclosure statement: The authors have no conflicts of interest to disclose.
Ethical standards: The Non-Biomedical IRB at the University of North Carolina at Chapel Hill approved this study (study #08-0176). Because the patient-level data were secondary data collected in 2004, participant consent was not required for this study.
References
1. Florida Center for Nursing. Types of nurses. Retrieved from http://www.flcenterfornursing.org/FAQ/TypesofNurses.aspx. Accessed 30 September 2014.
2. Bostick JE, Rantz MJ, Flesner MK, & Riggs CJ. Systematic review of studies of staffing and quality in nursing homes. Journal of the American Medical Directors Association 2006; 7: 366-376.
3. Castle NG. Nursing home caregiver staffing levels and quality of care – A literature review. Journal of Applied Gerontology 2008; 27(4): 375-405.
4. Collier E & Harrington C. Staffing characteristics, turnover rates, and quality of resident care in nursing facilities. Research in Gerontological Nursing 2008; 1(3): 157.
5. Kane RL. Commentary: Nursing home staffing – More is necessary but not necessarily sufficient. Health Services Research 2004; 39(2): 251-255.
6. Castle NG & Engberg J. The influence of staffing characteristics on quality of care in nursing homes. Health Services Research 2007; 42(5): 1822-1847.
7. Castle NG & Engberg J. Further examination of the influence of caregiver staffing levels on nursing home quality. Gerontologist 2008; 48(4): 464-476.
8. Zhang NJ, Unruh L, & Wan TTH. Gap in nurse staffing and nursing home resident needs. Nursing Economic$ 2013; 31(6): 289-297.
9. Stone R & Harahan MF. Improving the Long-term care workforce serving older adults. Health Affairs 2010; 29(1): 109-115.
10. Werner RM & Konetzka RT. Advancing nursing home quality through quality improvement itself. Health Affairs 2010; 29(1): 81-86.
11. Gaugler JE. The challenge of workforce retention in long-term care. Journal of Applied Gerontology 2014; 33(1): 3-5.
12. Munroe DJ. The influence of registered nursing home staff on the quality of nursing home care. Research in Nursing and Health 1990; 13: 263-270.
13. Spector WD & Takada HA. Characteristics of nursing homes that affect resident outcomes. Journal of Aging and Health 1991; 3(4): 427-454.
14. Harrington C & Swan JH. Nursing home staffing, turnover, and case mix. Medical Care Research and Review 2003; 60: 366-392.
15. Castle NG & Engberg J. Staff turnover and quality of care in nursing homes. Medical Care 2005; 43(6): 616-626.
16. Hyer K, Thomas KS, Branch LG, Harman JS, Johnson CE, & Weech-Maldonado R. The influence of nurse staffing levels on quality of care in nursing homes. The Gerontologist 2011; 51(5): 610-616.
17. Caffrey C. Potentially preventable emergency department visits by nursing home residents: United States, 2004, National Center for Health Statistics. 2010. http://www.cdc.gov/nchs/data/databriefs/db33.htm. Accessed 6 September 2014.
18. Ouslander JG & Berenson RA. Reducing unnecessary hospitalizations of nursing home residents. New England Journal of Medicine 2011; 365(13): 1165-1167.
19. Centers for Medicare & Medicaid Services. Initiative to Reduce Avoidable Hospitalizations Among Nursing Facility Residents. Retrieved from http://www.cms.gov/Medicare-Medicaid-Coordination/Medicare-and-Medicaid-Coordination/Medicare-Medicaid-Coordination-Office/ReducingPreventableHospitalizationsAmongNursingFacilityResidents.html. Accessed 6 September 2014.
20. Castle NG & Engberg J. Organizational characteristics associated with staff turnover in nursing homes. The Gerontologist 2006; 46(1): 62-73.
21. Stearns SC, Park J, Zimmerman S, Gruber-Baldini AL, Konrad TR, & Sloane PD. Determinants and effects of nurse staffing intensity and skill mix in residential care/assisted living settings. Gerontologist 2007; 47(5): 662-671.
22. Castle NG & Anderson RA. Caregiver staffing in nursing homes and their influence on quality of care using dynamic panel estimation methods. Medical Care 2011; 49: 545-552.
23. Shield R, Rosenthal M, Wetle T, Tyler D, Clark M, & Intrator O. Medical staff involvement in nursing homes: development of a conceptual model and research agenda. Journal of Applied Gerontology 2014; 33(1):75-96.